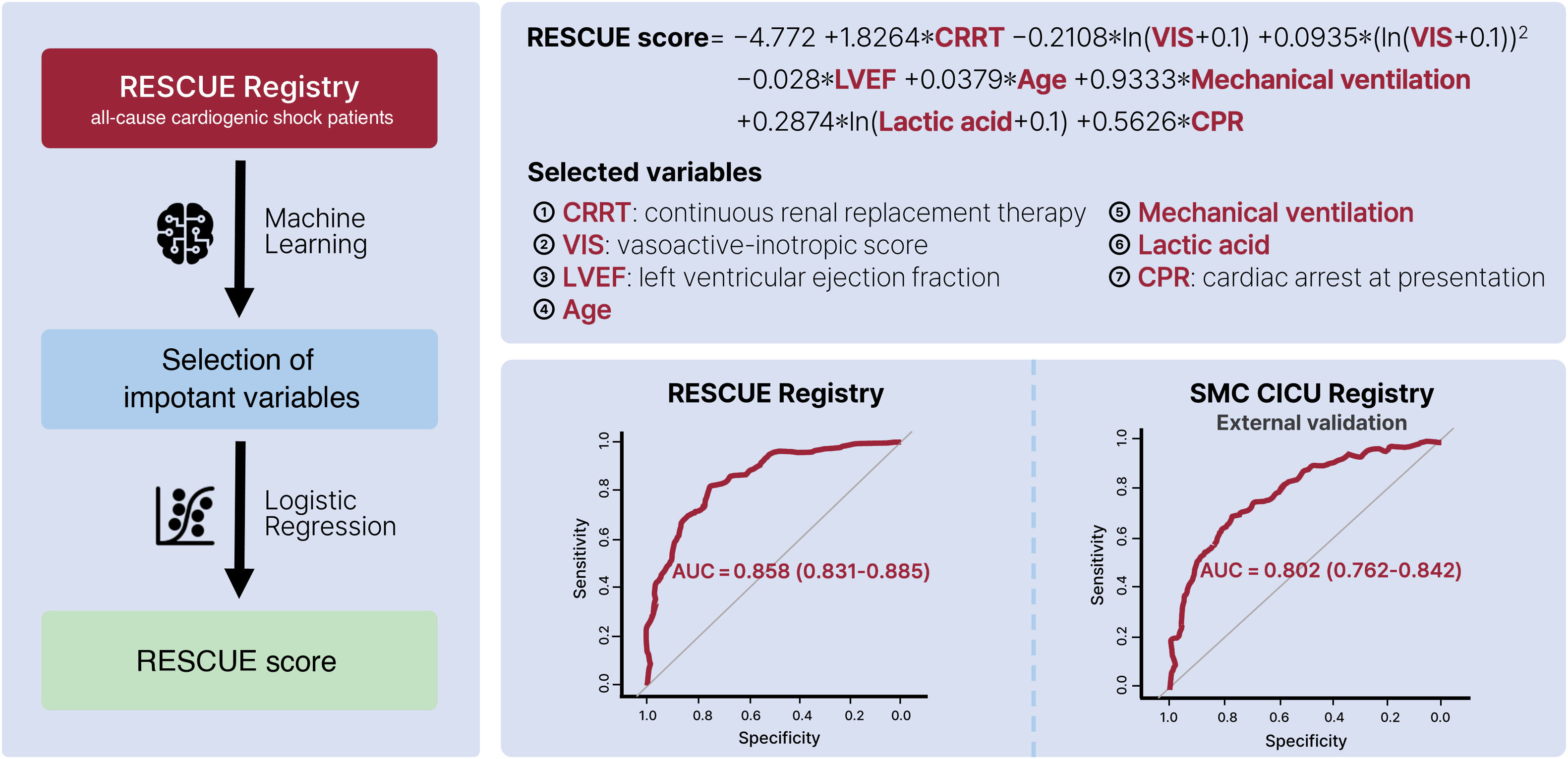
Despite advances in mechanical circulatory support, mortality rates in cardiogenic shock (CS) remain high. A reliable risk stratification system could serve as a valuable guide in the clinical management of patients with CS. This study aimed to develop and externally validate a risk prediction model for in-hospital mortality in CS patients using machine learning (ML) algorithms.
MethodsData from 1247 patients with all-cause CS in the RESCUE registry (January 2014-December 2018) were analyzed. Key predictive variables were identified using 4 ML algorithms. A risk prediction model, the RESCUE score, was developed using logistic regression based on the selected variables. Internal validation was conducted within the RESCUE registry, and external validation was performed using an independent CS registry of 750 patients.
ResultsThe 4 ML models identified 7 predictors: age, vasoactive inotropic score, left ventricular ejection fraction, lactic acid level, in-hospital cardiac arrest at presentation, need for continuous renal replacement therapy, and mechanical ventilation. The RESCUE score demonstrated strong predictive performance, with an AUC of 0.86 (95%CI, 0.83-0.88) for in-hospital mortality. Ten-fold internal cross-validation yielded an AUC of 0.86 (95%CI, 0.77-0.95). External validation showed an AUC of 0.80 (95%CI, 0.76-0.84).
ConclusionsOur ML-based risk-scoring system, the RESCUE score, demonstrated excellent predictive performance for in-hospital mortality in all patients with CS, regardless of cause. The system could be a useful and reliable tool to estimate risk stratification of CS in everyday clinical practice. Clinical trial registration: NCT02985008.
Keywords
Identify yourself
Not yet a subscriber to the journal?
Purchase access to the article
By purchasing the article, the PDF of the same can be downloaded
Price: 19,34 €
Phone for incidents
Monday to Friday from 9am to 6pm (GMT+1) except for the months of July and August, which will be from 9am to 3pm