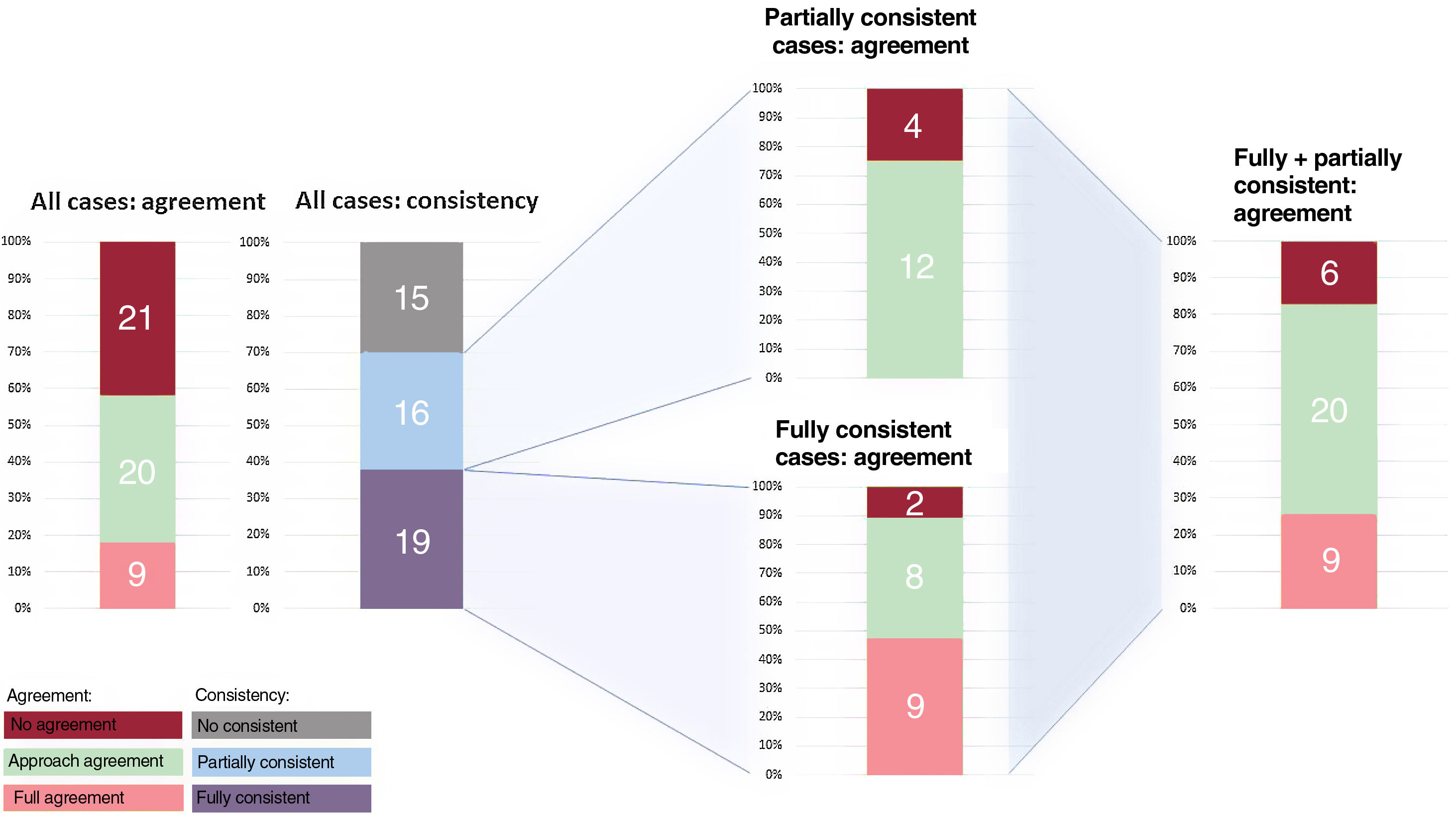
ChatGPT, an artificial intelligence dialogue-based language model, has generated strong expectations worldwide due to its surprising ability to convincingly answer complex queries formulated in plain natural language. It has been used in a wide variety of fields, including education, computer programming, and journalism, with potentially paradigm-shifting results. The medical community is no exception. ChatGPT has successfully passed the exams required to obtain a medical license,1 draft scientific abstracts,2 and compose complete medical reports.3 In cardiology, the bot has provided appropriate cardiology-related assistance for common cardiovascular conditions in simulated patients4 and has outperformed medical students in standardized cardiovascular tests.5
In light of the above, there is a strong temptation to try ChatGPT out as a decision-support tool in real-world clinical data. However, it is important to ask whether ChatGPT is able to process real-world medical records and suggest appropriate treatment. Most of the current literature focuses on its application in “synthetic” databases with highly preprocessed, curated texts, and/or multiple-choice answers.1–6 Real-world accuracy cannot be directly inferred from those settings. To answer this question, we assessed the agreement between ChatGPT and a heart team consisting of cardiologists and cardiac surgeons in a specific use case: the decision-making process in patients with severe aortic stenosis.
We performed a descriptive retrospective analysis of the medical records of 50 consecutive patients with aortic stenosis presented at a heart team meeting of our institution between January 1, 2022 and February 14, 2022 (these dates were chosen to guarantee that information on the patients’ eventual treatment was available). Depending on a wide variety of variables, the treatment of these patients consisted of the following options: a) surgical valve replacement; b) percutaneous valve implant; or c) medical treatment. The management strategies of the heart team were compared with those recommended by ChatGPT. An anonymized summary of each patient's status was produced by a cardiologist, who copy-pasted together the following sections from the electronic health record: demographics, past medical history, echocardiogram, coronary angiogram, symptoms, and diagnosis. During the second half of February 2023, this information was entered 3 times as a prompt in ChatGPT (GPT-3.5, 13 February 2023 version) as part of an enquiry about the optimal treatment. Initially, the question was “What is the best treatment for this patient?”, but the responses of ChatGPT were too comprehensive and included medications and interventions for any concurrent comorbidities in the test patient. Therefore, the final prompt used for the experiments was “What is the best treatment for the aortic stenosis in the following patient?” to elicit a focused response that would facilitate data interpretation, labeling, classification, and processing. No further changes to the prompt were necessary to obtain meaningful answers. Responses were codified as a) surgery; b) transcatheter aortic valve implantation (TAVI); c) medical treatment; d) undefined intervention (ChatGPT recommended aortic valve replacement but did not specify whether the approach should be surgical or percutaneous); or e) inconclusive. The results were classified according to the following definitions:
- -
Fully consistent: all 3 responses recommended exactly the same treatment.
- -
Partially consistent: all 3 responses recommended a similar approach (intervention vs medical treatment).
- -
Full agreement: fully consistent response that matched the heart team's assessment.
- -
Agreement on approach: fully or partially consistent response that matched the heart team's “intervention vs medical treatment” assessment.
Figure 1 shows the results in detail. The mean age was 78 years, and 41% were men. The heart team's decision was TAVI in 56%, surgery in 40%, and medical treatment in 4% of cases. Of 150 responses generated by ChatGPT, 14 (9%) were inconclusive. A total of 70% of ChatGPT's recommendations were at least partially consistent and 38% were fully consistent. There was agreement on approach in 58% of the cases but full agreement in only 18% of cases. Fifteen recommendations were inconsistent and 6 recommendations that were consistent diverged from the heart team's decision, representing a total of 21 errors. Of these 21 cases, 10 (48%) had other concomitant valve or coronary artery disease requiring intervention, 4 (19%) were cases in which the indications for intervention had a lower level of evidence, and 7 (33%) were cases of isolated symptomatic aortic stenosis. However, when the recommendations were fully consistent, ChatGPT showed at least agreement inapproach in 89% of the cases.
This study has some limitations, including its exploratory nature and small sample size. In addition, as a single-center experience, the gold standard was the decision of a particular heart team, which theoretically could have its own biases.
ChatGPT's treatment suggestions agreed with those of the medical experts in 58% of the cases. Agreement was low for specific treatments, and moderate for intervention vs medical treatment. Unsurprisingly, ChatGPT tended to agree with the heart team's decision in cases where it consistently provided similar answers to repeated instances of the same question. However, agreement and consistency were substantially impaired in clinically complex cases. These results were obtained using a system designed solely as a generic conversational bot with no specialized training in a highly challenging context (open-ended question, complex disease). There results could be markedly be improved by future versions specifically trained for medical decision support.
FUNDINGThis work has not received any specific funding.
ETHICAL CONSIDERATIONSDue to the retrospective nature of this work and the complete anonymization of data, the requirement to retrieve informed consent from patients was waived. The research protocol was reviewed and approved by Comité de Ética de la Investigación con Medicamentos del Área de Salud Valladolid Este with code PI 23-3194. The study design, methodology and data were analyzed for signs of any potential gender bias and none was found.
STATEMENT ON THE USE OF ARTIFICIAL INTELLIGENCEAs described in the text, ChatGPT 3.5 was used in the experiments of this work as subject of study. No IA-based tool whatsoever was used for manuscript writing or for data analysis or interpretation of the results.
AUTHORS’ CONTRIBUTIONSC. Baladrón, T. Sevilla and J.A. San Román designed the study; C. Baladrón and T. Sevilla designed and performed the experiments. C. Baladrón and J.A. San Román wrote the initial draft of the manuscript. M. Carrasco-Moraleja, I. Gómez-Salvador and J. Peral-Oliveira reviewed the data and methodology, produced the figures, and performed data analysis. All authors participated in manuscript reviewing and correction.
CONFLICTS OF INTERESTThe authors have no conflicts of interest to disclose.