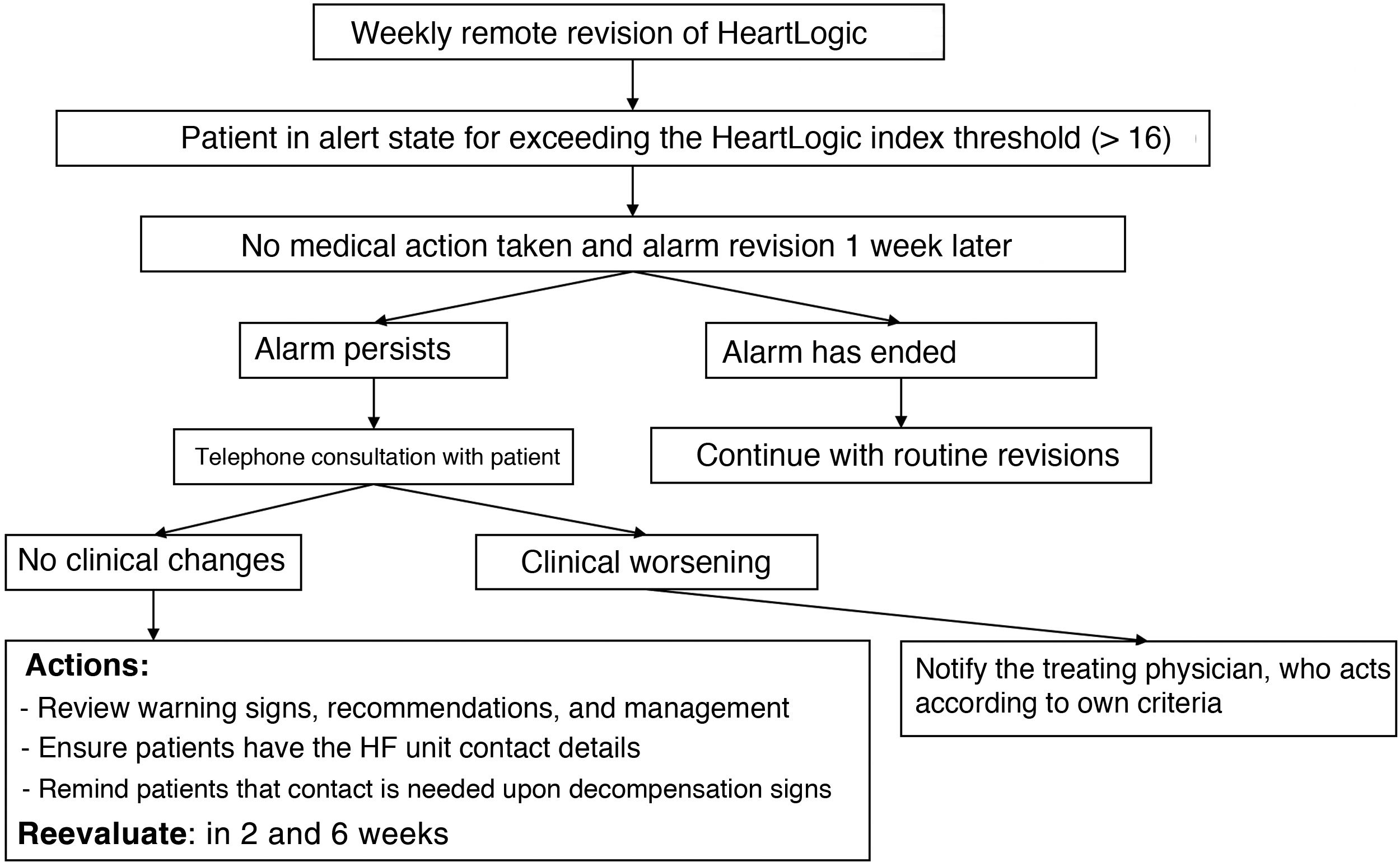
HeartLogic is a multiparametric algorithm incorporated into implantable cardioverter-defibrillators (ICD). The associated alerts predict impending heart failure (HF) decompensations. Our objective was to analyze the association between alerts and clinical events and to describe the implementation of a protocol for remote management in a multicenter registry.
MethodsWe evaluated study phase 1 (the investigators were blinded to the alert state) and phases 2 and 3 (after HeartLogic activation, managed as per local practice and with a standardized protocol, respectively).
ResultsWe included 288 patients from 15 centers. In phase 1, the median observation period was 10 months and there were 73 alerts (0.72 alerts/patient-y), with 8 hospitalizations and 2 emergency room admissions for HF (0.10 events/patient-y). There were no HF hospitalizations outside the alert period. In the active phases, the median follow-up was 16 (95%CI, 15-22) months and there were 277 alerts (0.89 alerts/patient-y); 33 were associated with HF hospitalizations or HF death (n=6), 46 with minor decompensations, and 78 with other events. The unexplained alert rate was 0.39 alerts/patient-y. Outside the alert state, there was only 1 HF hospitalization and 1 minor HF decompensation. Most alerts (82% in phase 2 and 81% in phase 3; P=.861) were remotely managed. The median NT-proBNP value was higher within than outside the alert state (7378 vs 1210 pg/mL; P <.001).
ConclusionsThe HeartLogic index was frequently associated with HF-related events and other clinically relevant situations, with a low rate of unexplained events. A standardized protocol allowed alerts to be safely and remotely detected and appropriate action to be taken on them.
Keywords
Heart failure (HF) is a public health challenge due to its high prevalence, clinical severity, and associated costs.1–5 Most HF patients experience decompensations that generally result in hospitalization.6 These episodes, in addition to increasing disease-related costs, have a negative impact on patients’ quality of life and prognosis.7 There is thus a need for new strategies that avoid decompensations or that enable early interventions and minimize hospitalizations.
Decompensation indicators that can be recognized by patients, such as weight gain and edema, appear soon before the need for emergency hospitalization, limiting their ability to guide interventions and avoid admission.8 Devices have thus been developed to facilitate the early detection of decompensations through the identification of pathophysiological changes developing weeks before hospitalization, such as elevated ventricular filling pressure, increased sympathetic tone, and decreased thoracic impedance.8
HeartLogic is an algorithm incorporated into some basic implantable cardioverter-defibrillators (ICDs) or cardiac resynchronization therapy (CRT)-ICDs from Boston Scientific (St Paul, United States) that measures and combines 5 parameters—heart sounds, thoracic impedance, respiratory volume and frequency, physical activity, and night heart rate—into a single numerical value. This information can be visualized through the LATITUDE remote monitoring platform. The MultiSENSE trial validated the use of HeartLogic in the early diagnosis of HF decompensation.9 However, because real-life experience with this tool is scarce,10–12 the present RE-HEART study was designed to obtain data on the clinical usefulness of HeartLogic.
METHODSRE-HEART is an observational, prospective, and retrospective multicenter registry that was approved by the Drug Research Ethics Committee (CEIm) of Hospital Universitario 12 de Octubre (approval number, 19/327).
Study populationThe present study included all patients older than 18 years with an ICD or CRT-ICD with incorporated HeartLogic and capability for remote monitoring through the LATITUDE platform, history of HF with reduced left ventricular ejection fraction (≤ 35%) at implantation, the ability to attend an outpatient service or provide the required information if managed in another center, with life expectancy longer than 1 year, and with provision of signed informed consent.
Study phasesIn September 2019, a 1-year prospective follow-up study (phase 3) was started, which included both patients previously implanted with a device and those with new devices. During this period, new patients were added from the centers and there was no minimum follow-up time. The standardized follow-up protocol used by all centers, which could be implemented by nursing staff, is shown in figure 1 and in .
Given that the LATITUDE platform allows revision of the historical record of HeartLogic index values from the previous year, a retrospective analysis was also performed, which could be applied for each patient and/or center to 2 situations: a) a period (phase 1) in which the patient had the device but the center lacked access to the platform to visualize the HeartLogic index, meaning that the blinded researchers could not act based on the HeartLogic index but could a posteriori review its values, and b) a period (phase 2) in which the center had activated the platform to visualize the HeartLogic index and acted according to the local protocol.
EventsThe same criteria as those applied in the MultiSENSE study9 were used to define the events of interest. Thus, HF decompensations were classified as follows:
- •
Major: HF hospitalization, comprising an unscheduled visit requiring intravenous treatment or resulting in HF death.
- •
Minor: clinical data of acute disease exacerbation that, based on clinician criteria, required modification of HF treatment.
HeartLogic alerts were classified as follows:
- •
True: when the alert began (the moment at which the predefined HeartLogic index of 16 was exceeded) before the HF decompensation. When the alert had finalized before the decompensation, the interval between the alert and decompensation had to be < 30 days.
- •
Explained: when relevant clinical conditions were identified (eg, dietary or medication indiscretion, decreased resynchronization percentage, onset of atrial fibrillation, anemia, infection) that could produce HF decompensation but with no data on this at the time of assessment. The onset of the alarm period of the HeartLogic index could be before or after the identified cause and was established at a maximum interval of±30 days.
- •
Unexplained: when none of the above-mentioned criteria were met.
Also recorded were HF events not associated with an alert in the HeartLogic index (false negatives).
In addition, to compare the diagnostic accuracies of N-terminal pro-B type natriuretic peptide (NT-proBNP) and of the HeartLogic index for confirming or ruling out HF decompensation, NT-proBNP was measured at baseline and at decompensation.
Statistical analysisNormally distributed continuous variables are shown as mean±standard deviation while nonnormally distributed variables are shown as median [interquartile range]. Distribution normality was tested using the Kolmogorov-Smirnov test. Categorical variables are expressed as percentages.
Differences in proportions were compared using the chi-square test. All statistical analyses were performed with R version 4.0.2 (The R Foundation for Statistical Computing Platform, Austria).
RESULTSIn total, 288 patients from 15 Spanish centers were enrolled between September 2019 and September 2020 (see the complete list of participating hospitals in the ). The patients’ baseline characteristics are shown in table 1.
Patients’ baseline characteristics
Patients, n | 288 |
Male sex | 222 (77) |
Age, y | 68±10 |
Educational level | |
Without schooling | 25 (9) |
Basic education | 144 (50) |
High school or vocational training | 91 (32) |
University | 28 (10) |
Living situation | |
Family | 260 (90) |
Care home | 1 (1) |
Alone | 25 (9) |
Shared residence | 1 (1) |
Dedicated carer | 66 (23) |
Body mass index | 28±4 |
Ischemic heart disease as cause of LV dysfunction | 151 (52) |
NYHA functional class | |
I | 47 (16) |
II | 166 (58) |
III | 75 (26) |
LVEF <35% | 217 (75) |
Comorbidities | |
History of atrial fibrillation | 112 (39) |
Atrial fibrillation at implantation | 66 (23) |
Hypertension | 214 (74) |
Diabetes | 116 (40) |
Dyslipidemia | 169 (59) |
Smoking | 175 (64) |
Smoker | 31 (11) |
Exsmoker | 144 (53) |
COPD | 48 (17) |
Chronic kidney disease | 77 (27) |
On hemodialysis | 5 (2) |
Previous stroke | 31 (11) |
SAHS | 33 (11) |
Medical therapy | |
Beta-blockers | 274 (95) |
ACEIs, ARBs, or valsartan/sacubitril | 265 (92) |
Valsartan/sacubitril | 145 (50) |
MRAs | 215 (75) |
Diuretics | 207 (72) |
Amiodarone | 64 (22) |
Ivabradine | 35 (12) |
Analytical data | |
NT-proBNP, pg/mL | 1234 [626-2425] |
Creatinine, mg/dL | 1.27±0.82 |
Glomerular filtration rate, mL/min | 63±22 |
Devices implanted | |
CRT-ICD | 234 (81) |
ICD in primary prevention | 241 (84) |
ACEIs, angiotensin-converting enzyme inhibitors; ARBs, angiotensin II receptor blockers; COPD, chronic obstructive pulmonary disease; CRT, cardiac resynchronization therapy; ICD, implantable cardioverter-defibrillator; LV, left ventricular; LVEF, left ventricular ejection fraction; MRAs, mineralocorticoid receptor antagonists; NT-proBNP, N-terminal pro-B-type natriuretic peptide; NYHA, New York Heart Association; SAHS, sleep apnea-hypopnea syndrome.
Values represent No. (%), mean ± standard deviation, or median [interquartile range].
Of these, 101 patients participated in phase 1, with a mean follow-up period of 10 (95% confidence interval [95%CI], 5-19) months; 94 patients participated in phase 2, with a mean follow-up duration of 7 (95%CI, 3-10) months, and 267 patients participated in phase 3, with a mean follow-up duration of 13 (95%CI, 11-13) months. The median follow-up of the 2 combined active phases (phases 2 and 3) was 16 (95%CI, 15-22) months.
Blind study phase (phase 1)The HeartLogic index crossed the threshold 73 times in 39 patients (0.72 alerts/patient-y). The time in alert was 8% of the follow-up period. During this phase, 9 patients experienced 8 hospitalizations and 2 emergency room admissions for HF (0.10 events/patient-y).
These events were associated with the HeartLogic alert state, with a rate of events within the alert state of 1.23/patient-y. Other events associated with the alerts were 3 unscheduled consultations for HF decompensations and 7 hospitalizations for reasons other than HF. In the 53 remaining alerts, no related clinical events were detected, which is why they were considered unexplained (0.52 alerts/patient-y). However, there were no HF hospitalizations outside the HeartLogic alert state.
Events detected during the active study phases (phases 2 and 3)During these 2 phases (figure 2), which were analyzed together, 277 HeartLogic alerts were reported in 136 patients (0.89 alerts/patient-y). The time in alert state was 11% of the follow-up period. Because 33 HeartLogic alerts were associated with hospitalizations (27 for HF and 6 HF deaths) and 46 were unscheduled consultations (in-person or telephone) due to HF decompensation, there were 79 true alerts. There were 0.11 major HF events/patient-y. The mean time from alert onset to HF hospitalization was 20±15 days.
Alerts and events detected during the active phases of the study (phases 2 and 3). Unexplained alerts were those that could not be linked to a relevant clinical event. Explained alerts were those linked to clinical events of interest, such as dietary/medication indiscretions or lifestyle changes, but that lacked clear data on HF at that time. HF, heart failure; VF, ventricular fibrillation; VT, ventricular tachycardia.
Although 78 explained alerts were associated with other events, no explanation was found for 120 alerts (0.39 alerts/patient-y).
Outside the alert state, there was only 1 HF hospitalization and 1 unscheduled consultation for HF decompensation.
The HeartLogic diagnostic indices in both periods are shown in table 2.
HeartLogic diagnostic index values in the different phases of the study
Phase 1 (blind) | Phases 2 and 3 (active) | |
---|---|---|
Sensitivity | 100% | 98% |
Specificitya | 93% | 90% |
Positive predictive valueb | 18% | 29% |
Positive predictive valuec | 27% | 57% |
Negative predictive valued | 100% | 99.9% |
Rate of unexplained alerts | 0.52 alerts/patient-y | 0.39 alerts/patient-y |
Rate of false-positive alertse | 0.59 alerts/patient-y | 0.64 alerts/patient-y |
HF, heart failure.
According to the definition of the MultiSENSE study9: true negatives/(true negatives + false positives) during periods without heart failure (excluding periods from 30 days before to 15 days after a HF event). Days were classified individually as true negative if the HeartLogic index was not in an alert state and as false positives if it was in an alert state.
According to the definition of the MultiSENSE study9: true negatives/(true negatives + false negatives).
Of the 44 HeartLogic alerts reported in 32 patients during phase 2, 32 (73%) resulted in a consultation (in-person or telephone). During phase 3, consultations were more frequent, comprising 198 of the 233 alerts (85%, P=.047) in 130 patients.
The alerts prompted medical acts (treatment modifications or educational interventions) in 27% of cases during phase 2 and in 39% during phase 3 (P=.138).
There were 4 HF hospitalizations (0.07 events/patient-y) in phase 2 and 23 (0.09 events/patient-y) in phase 3 (P=.724).
Of the 44 alerts in phase 2, 36 (82%) did not require in-person visits and could be remotely managed. During phase 3, remote consultations were used to manage 188 of the 233 alerts (81%; P=.861). The total numbers of telephone contacts with patients were 35 (0.65 contacts/patient-y) in phase 2 and 287 (1.12 contacts/patient-y) in phase 3 (P=.002).
The estimated workload for reviewing and managing the HeartLogic alerts (table 3), once the staff responsible was trained, was 1 hour per week for following up 30 patients in a center.
Management of alerts during the active phases and related events
Phase 2 (7 mo) | Phase 3 (13 mo) | P | |
---|---|---|---|
All alerts, alerts/patient-y | 44 (0.81) | 233 (0.91) | .509 |
Remote management of alerts | 36 (82%) | 188 (81%) | .861 |
Annual number of telephone contacts per patient | 0.65 | 1.12 | .002 |
Alerts requiring action | 12 (27%) | 91 (39%) | .138 |
Actions performed | |||
Adjustment of diuretics or other drugs | 11 (92%) | 75 (82%) | .685 |
Changes in device programming | 1 (8%) | 13 (14%) | .999 |
Reinforcement of patients’ HF education | 1 (8%) | 6 (7%) | .591 |
Readmission to hospital for reason other than HF | 2 (25%) | 10 (11%) | .629 |
Cardioversion | - | 4 (4%) | .999 |
CPAP | - | 2 (2%) | .999 |
AVN ablation | - | 1 (1%) | .999 |
Events | |||
Minor HF decompensation | 7 | 39 | |
HF hospitalization | 4 | 23 | |
Death from HF | - | 6 | |
Hospitalization from other causes | 3 | 7 | |
Death from other causes | 1 | 3 |
AVN, atrioventricular node; CPAP, continuous positive airway pressure; HF, heart failure.
In total, 194 measurements of plasma NT-proBNP levels were performed, 32 during a HF decompensation event (figure 3).
Scatter plot of NT-proBNP levels and HeartLogic alerts. In total, 94% of HF decompensations were associated with an elevated HeartLogic index, whereas only 6% of stable patients were in an alert state (P < .001; specificity, 94%). The median NT-proBNP value was higher in patients in a HeartLogic index alert than in those outside of an alert (7378 vs 1210 pg/mL; P < .001). The median NT-proBNP value was higher during decompensations than in the stable phase (8624 vs 1213 pg/mL; P < .001). HF, heart failure; NT-proBNP, N-terminal pro-B-type natriuretic.
The median NT-proBNP levels were 8624 [2592-19 503] pg/mL at decompensation and 1213 [597-2350] pg/mL (P < .001) under stable conditions.
NT-proBNP levels were measured 39 times when the HeartLogic index was in an alert state. The median NT-proBNP levels within and outside of alerts were 7378 [2214-19 377] and 1210 [599-2276] pg/mL, respectively (P < .001).
DISCUSSIONThe main finding of this study was the ability of HeartLogic to remotely identify HF events and other clinical situations in a real-life clinical setting.
In phase 1, the event rate during the alert state was 12 times higher than that seen in the entire period (0.10 vs 1.23 events/patient-y), indicating higher vulnerability to decompensations in this period. During phases 2 and 3, more than half of the alerts were related to HF decompensation or had an explanation. The low rate of HF events is in line with the findings of other studies9,11,12 and can be explained by the high percentage of prognostic treatments and devices in our population.
In addition, the rates of unexplained alerts were 0.52/patient-y in phase 1 and 0.39 in the prospective phases, lower than that reported in the MultiSENSE trial (1.47). This can partly be explained by our inclusion of minor HF decompensation events and other relevant clinical events. We decided to include minor decompensations in the analysis. Such decompensations were managed on an outpatient basis, given that this is a frequent approach in Spain, often with the support of the day hospital. At the same time, the ability of HeartLogic to identify other clinical situations is valuable. Such events included medication or dietary indiscretions, respiratory infections, and onset of atrial fibrillation, which may trigger HF decompensation and require intervention and strict monitoring until their resolution.
In agreement with other studies of remote monitoring, adjustment of diuretic therapy was the most frequent clinical action. Nonetheless, the possibility of reviewing the overall functioning of the device and arrhythmic events in the LATITUDE platform enables the performance of other interventions, such as programming modifications, cardioversion of atrial arrhythmias, and atrioventricular node ablation.
We obtained high sensitivity and negative predictive values, reflecting the low probability of HF decompensations outside the alert state. In our study, outside the alert state, there were no hospitalizations in phase 1 and only 1 hospitalization and 1 consultation for HF decompensation in the active phase. Similarly, the specificity in both periods was high. However, the positive predictive value was lower, which was partly influenced by the low rate of HF events.
These data indicate that HeartLogic is useful for the remote monitoring of HF patients, particularly in situations such as that generated by the COVID-19 pandemic, both due to its capacity for the early detection of decompensation and for determining the stability of patients outside alert states. These properties permit less frequent follow-ups, minimize unnecessary contact with hospitals, and limit costs while patients are outside states, given the low probability of events. Another valuable finding of our study is that more than 80% of alerts could be managed by telephone.
The 15 participating centers represent a broad spectrum of Spanish territory. In light of these data, the units deciding to apply the HeartLogic index to the management of their patients were able to assess the use of the treatment algorithm used in RE-HEART, given that the present study constitutes a real-life clinical validation of the reported utility rates.
The possibility of reviewing the HeartLogic index value from up to 1 year before allowed us to study its relationship with HF decompensations in a unique setting, in which the clinical staff were blind to the algorithm values and could thus not act accordingly (phase 1). After the “unblinding” of HeartLogic, the data were differentiated into a phase in which the center acted according to a local protocol (phase 2) or according to the standardized protocol (phase 3) for 1 year.
In the MultiSENSE trial, the mean time from HeartLogic alert onset to HF event was 34 days (in our study, 20±15 days), which is why it was considered prudent to establish a weekly review of the HeartLogic index. This schedule represents a reasonable workload, estimated at 1 hour per week for 30 patients under follow-up, and, although phase 3 provoked a higher number of calls than phase 2, resulted in slightly more than 1 call per patient per year. In addition, the time in alert state in our study was just 8% in phase 1 and 11% in phases 2 and 3, lower than the 17% reported in the MultiSENSE trial.
The possibility of predicting a HF decompensation event or detecting it early enough to enable an intervention and avoid hospitalization is appealing. It is not surprising that technological advances can boost the management of HF patients. The initial experiences with sensors incorporated into ICDs to identify the early signs of decompensation were disheartening due to their low sensitivity (20%-34%).13–17 However, a multiparametric assessment could improve the performance of decompensation detection systems.
The MultiSENSE trial validated the HeartLogic algorithm, which incorporates various variables detected by ICDs (with or without CRT) with a sensitivity of 70%, an acceptable rate of unexplained alerts (1.47/patient-y), and a negative predictive value close to 100%. Some of the advantages of HeartLogic are that it does not require implantation of a new device (which eliminates the associated risks and costs) or the active involvement of patients or their training and that its multiparametric character “powered” by 5 HF-related variables provides continuous and individualized information.9 Recent data indicate that HeartLogic use can reduce HF hospitalizations and decrease disease-related costs.12
Finally, although it has been reported that decompensation risk is higher in patients in an alert state and with baseline NT-proBNP levels > 1000 pg/mL,18 this is the first study to indicate that NT-proBNP levels can be significantly higher in HeartLogic alerts than outside alerts, which supports the belief that this is a period of higher decompensation risk.
LimitationsThe present work has the typical limitations inherent to observational studies. In addition, the retrospective character of phases 1 and 2 may have led to the underdetection of minor HF decompensation events, although this would strengthen the overall results obtained.
It cannot be ruled out that, during the active phase, the call from the health care team upon the detection of the onset of an alert state may have sometimes influenced the associated event. However, these events were allocated when the objective tests agreed and, in the case of HF decompensation, only if treatment modification was necessary. An overreaction may also have been generated in connection with the alert in this period. In addition, the estimated sensitivity may have been reduced as a result of an early intervention that possibly avoided a HF decompensation event.
Although the study protocol requested that researchers measure NT-proBNP levels at baseline and decompensation, they were only obtained from some patients. Accordingly, the related analysis must be interpreted with caution.
Obviously, these results were obtained from selected patients who had an ICD or CRT-ICD device with integrated HeartLogic algorithm. Nonetheless, the high number of participating centers and patients reflects a broad spectrum of the HF population in Spain.
Finally, although this study strengthens the value of HeartLogic as a useful tool for the identification of imminent HF decompensation, determination of its prognostic impact requires analysis of a large sample and assessment of the results of targeted clinical trials.
CONCLUSIONSThe real-life clinical practice experience of a diverse group of Spanish centers with HeartLogic use revealed a good correlation with HF decompensation events, consistent with elevated levels of NT-proBNP during alerts and other relevant clinical conditions, and a low rate of unexplained alerts. The data indicate that HF decompensation is highly unlikely outside alert states.
Most alerts can be resolved by telephone and the most frequent action was adjustment of diuretic therapy.
- -
HeartLogic is an algorithm available in some ICDs and CRT-ICDs that helps to predict HF decompensation about 1 month in advance. The reported rate of inappropriate alerts is 1.47 per patient-year. Patients who are in a HeartLogic alert with NT-proBNP > 1000 pg/mL have a higher risk of HF decompensation.
- -
The RE-HEART registry is a large clinical study of HeartLogic in clinical practice. The registry reflects the experience of 15 centers and 288 patients collected prospectively for 1 year with a shared protocol and retrospectively for 1 year. More than half of the detected alerts identified an imminent HF decompensation event or other relevant clinical problems, more than 80% of which could be resolved by telephone. The inappropriate alert rate was low and decompensations were rare outside of alerts. We report for the first time higher NT-proBNP values during a HeartLogic alert than outside of an alert.
None.
AUTHORS’ CONTRIBUTIONSJ. de Juan Bagudá and R. Salguero Bodes designed the study, participated in the data analysis, and drafted the original manuscript. J.F. Delgado Jiménez helped in the final drafting of the manuscript. All authors contributed to patient inclusion in the database and critically reviewed and approved the final manuscript.
CONFLICTS OF INTERESTJ. de Juan Bagudá declares fees for consultancy work and presentations from Boston Scientific. R. Cózar León declares fees for consultancy work from Boston Scientific. F. Arribas Ynsaurriaga declares fees for consultancy work and presentations from Boston Scientific, Medtronic, and Abbott. R. Salguero Bodes declares fees for consultancy work from Boston Scientific. The remaining authors have no conflicts of interest to declare.
The authors appreciate the help of Noelia de la Torre, Carlos Briz, Monica Campari, and Sergio Valsecchi with the study design, data interpretation, and critical revision of the article.
Supplementary data associated with this article can be found in the online version available at https://doi.org/10.1016/j.rec.2021.09.015