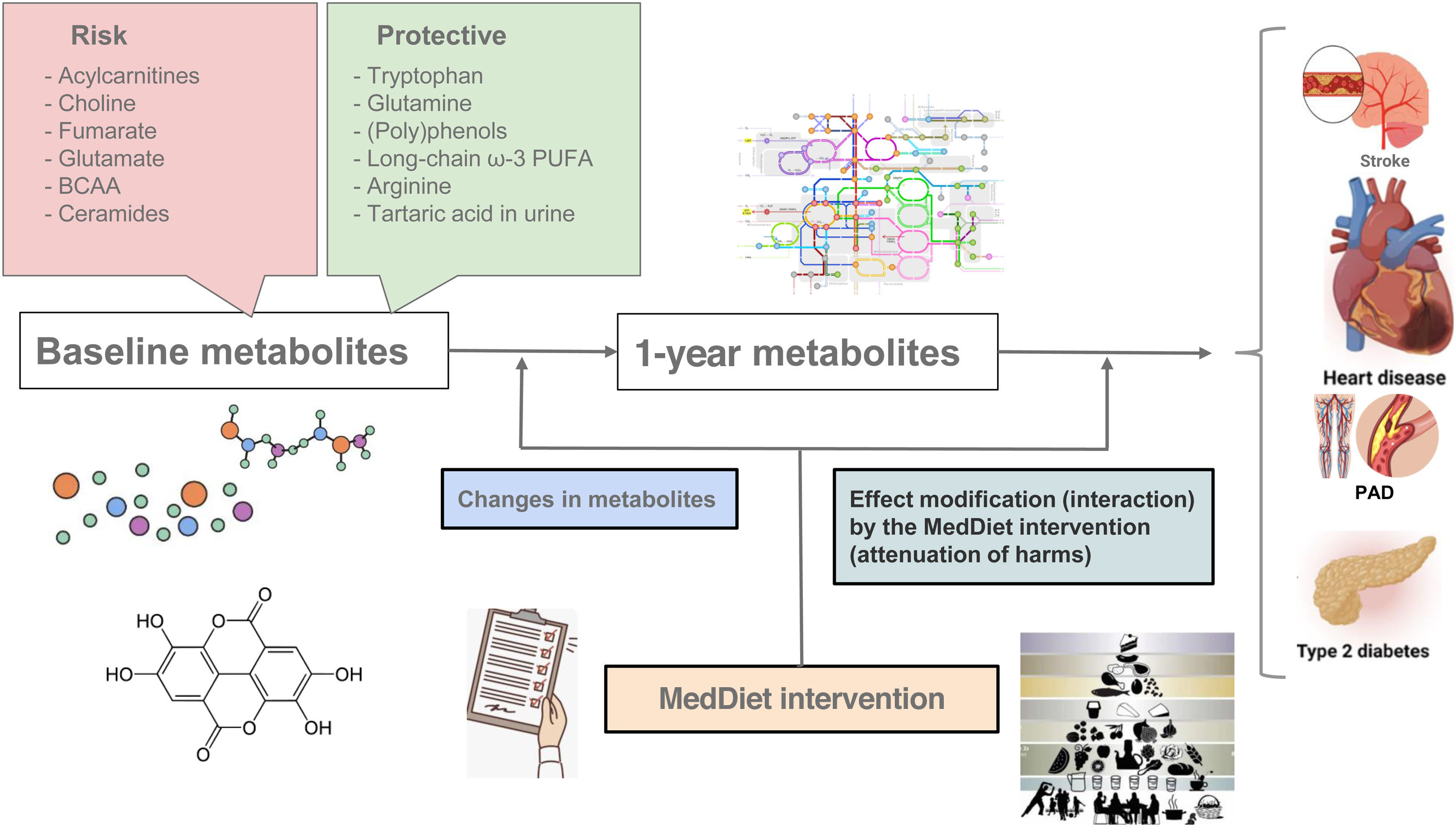
A growing body of research on nutrition omics has led to recent advances in cardiovascular disease epidemiology and prevention. Within the PREDIMED trial, significant associations between diet-related metabolites and cardiovascular disease were identified, which were subsequently replicated in independent cohorts. Some notable metabolites identified include plasma levels of ceramides, acyl-carnitines, branched-chain amino acids, tryptophan, urea cycle pathways, and the lipidome. These metabolites and their related pathways have been associated with incidence of both cardiovascular disease and type 2 diabetes. Future directions in precision nutrition research include: a) developing more robust multimetabolomic scores to predict long-term risk of cardiovascular disease and mortality; b) incorporating more diverse populations and a broader range of dietary patterns; and c) conducting more translational research to bridge the gap between precision nutrition studies and clinical applications.
Keywords
Metabolomics is the systematic study of the chemical transformations involving small-molecule metabolites within a cell or organism.1 Analyzing individual metabolomic profiles allows assessment of the metabolomic fingerprints associated with different dietary exposures. Metabolomics provides useful insights into the metabolic pathways linking dietary exposures and the risk of chronic diseases, including type 2 diabetes (T2D) and cardiovascular disease (CVD). It also contributes to the development of new hypotheses for disease prevention and treatment, following the paradigm of personalized medicine and nutrition.2 This approach was significantly advanced by a 2015 paper that introduced an algorithm for determining the optimal intake of postprandial glucose based on individual characteristics.3 Subsequent research has explored between-individual variations in metabolic responses.4 Recent studies further support the potential of precision nutrition to promote optimal cardiovascular health.5
This narrative review discusses recent advances in precision nutrition techniques and methods, their implications for cardiometabolic disease epidemiology and prevention, and future research directions.
METHODOLOGICAL ASPECTSMetabolomics, metabotyping, and machine learning methodsAdvanced computational modeling, particularly penalized machine learning models, that integrate multidimensional data—including diet, lifestyle, and omics—enables robust evidence supporting valid associations between diet and CVD risk. Multimetabolite signatures, which capture overall dietary patterns, can predict long-term risk of major CVD events beyond traditional risk factors. These metabolomic signatures reflect both dietary intake and the body's homeostatic responses to dietary exposures. Since metabolomic data in body fluids are objectively measured, they overcome some limitations of self-reported dietary intake.
Metabolomics analyses are instrumental in identifying novel metabolic pathways that underlie the relationships between diet and CVD outcomes. A seminal study in the PREDIMED trial developed a multimetabolite signature consisting of 67 plasma metabolites, which was replicated in 3 large cohorts in the United States (Nurses’ Health Studies I and II and the Health Professionals Follow-up Study [NHS/NHS2/HPFS]). This signature was strongly correlated with Mediterranean diet (MedDiet) adherence and was prospectively associated with CVD in both US and Spanish cohorts, even after adjusting for self-reported MedDiet adherence,6 thus showing huge potential for predicting long-term CVD risk.
Metabolomics methodsMetabolites can be studied using various analytical techniques. Targeted metabolomics involves measuring a selected group of pre-defined metabolites (eg, amino acids, lipids, sugars, and other small molecules). In contrast, nontargeted metabolomics approaches involve global profiling of the metabolome with a hypothesis-generating approach, including biomarker discovery. One extensively used method is liquid chromatography-mass spectrometry (LC-MS), which can be applied in both targeted and nontargeted approaches. By employing tandem mass spectrometry (MS/MS), fragmentation spectra are generated, providing structural information on unknown molecules. These data facilitate machine learning-based predictions of possible molecular formulas. The predicted structures are then arranged in a list based on likely matchups and confirmed using reference standards.7,8
Identifying metabolomic profiles enables the association of these profiles with incident CVD or T2D. This is frequently assessed in studies nested within the PREDIMED trial, using thousands of biospecimens from multiple cohorts for replication.6,9–13 One of the biggest challenges in metabolomics is identifying unknown metabolites associated with diet or disease, as there are still many gaps in understanding the degradative fate of important metabolites.
To address these gaps, researchers use computational chemistry to exploit enzyme promiscuity—the ability of enzymes to catalyze unexpected side reactions beyond their main function—and propose novel reactions not yet registered in databases.14,15
In the Jackson Heart Study cohort, LC-MS metabolomics analyses identified 124 known and unknown metabolites associated with incident T2D, including a potential linkage between lower serine and elevations in alanine and deoxyceramides. Additionally, the research revealed associations between diet-derived metabolites and disease-associated gene variants, shedding light on genes related to diseases such as heart failure (HF) in the elderly.12,16,17
MetabotypingMetabotyping involves phenotyping the metabolic processes of individuals, groups, or populations to better understand their metabolomic profiles and how these profiles relate to disease risk or response/nonresponse to interventions.18 Metabotypes have mostly been identified using biochemical and clinical data, but tools for integrating omics data are still lacking. Omics technologies can identify and characterize meaningful metabotypes through both static and dynamic methods. In dynamic studies, tensor decomposition methods like CANDECOMP/PARAFAC have been used to analyze dietary inputs, such as those from a beef diet, allowing for the prediction of metabotypes.19 This method enables a reverse-engineering approach to determine which diets can achieve specific adherence to certain metabotypes. Tailoring dietary macronutrient composition to insulin resistance phenotypes can significantly improve risk markers, highlighting precision nutrition as a feasible alternative to general dietary guidelines.20,21
Interactions between diet, microbiome, and host genetics are crucial.22 For example, a significant increase in postprandial plasma butyrate levels was observed after a 2-month intervention with the MedDiet, and plasma butyrate was correlated with insulin sensitivity.23 Further research is needed in individuals with different metabolite phenotypes and enterotypes. Saliva omics, though underused, have shown promise in linking diet and lifestyle with disease. Saliva composition is strongly influenced by age and oral microbiome diversity, but not by body mass index.24
Metabolic networks and the microbiotaMetabolic networks are computational models representing metabolic processes, encompassing thousands of internal reactions with functional and taxonomic annotations. The gut microbiota is likely to play a critical role in CVD pathophysiology.25 Metabolic networks are particularly interesting for assessing the composition and function of gut microbiota, which may mediate the effects of diet on CVD. The first comprehensive analysis of gut microbiota and its functional characteristics was conducted by AGORA, which initially studied 773 strains.26 Subsequently, the analysis was expanded to 7302 strains (AGORA2).27 AGREDA, a project developed at Tecnun (University of Navarra), extended AGORA by focusing on diet-related degradation pathways, specifically the degradation of polyphenols, demonstrating superior performance in predicting diet-specific output metabolites from gut microbiota compared with current reconstructions.28
Standard functional analysis involves integrating gut microbiota metabolic networks and bacterial compositional data to derive an activity score for reactions and metabolic subsystems. A bioinformatic tool that enables this task is q2-metnet,29 which can predict an individual's capacity to metabolize different compounds.
A more advanced strategy is steady-state analyses.30 In this approach, each taxon is treated as a distinct compartment within the system, together with an extracellular compartment where all inputs and outputs are examined. Each individual is then contextualized based on their gut abundance, as determined by 16S sequencing, and the availability of dietary compounds. The primary outcome is the prediction of microbial metabolite output, based on constraint-based optimization models, although it assumes a constant quantity of bacteria in the gut.
Dynamic metabolic models address the limitations of the steady-state assumption by considering the variation in the bacterial composition and including regulatory effects such as nutrient-microbe and microbe-microbe interactions. These models integrate agent-based modeling, constraint-based modeling, and machine learning with Bayesian systems. This approach can predict how different metabolites may alter the relative abundance of various taxa, although further developments are required.30
Food-based biomarkersThe Dietary Biomarker Development Consortium (DBDC) is a major initiative for food-based biomarker discovery and validation using metabolomics.31 The consortium is composed of 3 clinical centers (University of California, Davis; Harvard T.H. Chan School of Public Health; and the Fred Hutchinson Cancer Research Center, all in the USA) and a Data Coordinating Center (Duke University, USA). The DBDC is currently conducting systematic controlled feeding studies to identify blood and urine metabolite patterns associated with commonly consumed foods in the US population. These metabolites will be validated in free-living populations. The overarching goal is to create a comprehensive catalog of sensitive, specific, and robust plasma and urine metabolites for commonly consumed foods in the United States Department of Agriculture MyPlate. These biomarkers can be used in future observational studies and trials to assess dietary intakes, monitor adherence, and evaluate intervention targets aimed at improving diet quality.
Multiple determinants of metabolomic signatures for dietary patternsMultimetabolite signatures of dietary patterns reflect the combined effects of dietary intakes, biological responses, and other metabolic-affecting factors. Studies integrating all these aspects are likely to show stronger predictive ability than any partial assessment.32 In this context, blood metabolomic signatures have been analyzed for their adherence to 8 dietary patterns, including guideline-recommended diets (MedDiet, Alternative Healthy Eating Index, Dietary Approaches to Stop Hypertension), provegetarian or plant-based diets, and mechanism-driven diets such as the empirical dietary inflammatory pattern, and the empirical dietary index for hyperinsulinemia.33 These signatures have been shown to reproducibly correlate with the corresponding dietary index in diverse populations with varying age, sex, race, and dietary habits, using different technical platforms. Their associations with incident T2D have been replicated in multiple cohorts.
METABOLOMICS AND MEDITERRANEAN DIETPlasma metabolites and cardiovascular disease in PREDIMEDPREDIMED is a Spanish multicenter randomized trial (2003-2010) that evaluated the effects of the MedDiet for primary CVD prevention. Detailed information has been published elsewhere.34 Briefly, 7447 participants aged 55 to 80 years at high CVD risk but without overt disease were allocated to 1 of 3 dietary interventions: a) MedDiet supplemented with extra-virgin olive oil; b) MedDiet supplemented with mixed nuts; or c) a control group receiving a low-fat diet. The primary endpoint was major CVD (nonfatal myocardial infarction, nonfatal stroke, or cardiovascular death). Preintervention blood samples and samples at 1 year were collected after an overnight fast. EDTA-plasma aliquots were stored at −80°C.
The first case-cohort study (2013-2017) nested within the PREDIMED trial assessed plasma metabolites both at baseline and after 1 year of follow-up in 232 patients with the primary CVD endpoint and a random subcohort of 783 participants (figure 1). The continuation of this grant (2017-2021) included a series of 3 case-control studies assessing different CVD endpoints: atrial fibrillation (473 cases), HF (214 cases), and peripheral artery disease (169 cases). The currently ongoing continuation (2022-2026) consists of a case-cohort study with extended follow-up (11 years), including 606 cases of CVD (myocardial infarction, stroke, HF, or death from CVD causes) and a random subcohort of 679 participants, with a focus on urinary metabolites, particularly phenolic compounds.35–38 This study will contribute to the development of a multifluid (urine and plasma) multimetabolite signature predicting CVD risk.
Central illustration. Several National Institutes of Health-funded substudies nested within the PREDIMED randomized trial were designed to better understand the health benefits of MedDiet interventions on cardiometabolic risk prevention through metabolomics profiling. Case-cohort designs with repeated metabolomic profiling (baseline and after 1 year) allowed testing: a) the effects of baseline metabolites on CVD events; b) the effect of the intervention (MedDiet) on 1-year metabolite changes; c) the effects of changes in metabolites (from baseline to 1-year) on subsequent CVD events (after 1 year); d) the effect modification (potential attenuation) by the MedDiet intervention (interaction) on the associations between plasma metabolites and CVD events. All these tests were also applied to the risk of type 2 diabetes with analogous designs.
BCAA, branched-chain amino acids; CVD, cardiovascular disease; MedDiet, Mediterranean diet; PAD, peripheral artery disease; PUFA, polyunsaturated fatty acids.
In addition to studies on metabolomics and T2D, key findings in metabolomic studies for CVD nested in PREDIMED have been developed during the past few years due to a 10-year multidisciplinary collaboration of the Spanish PREDIMED teams (Principal Investigators at the Universities of Navarra, Rovira i Virgili, and Barcelona, Spain), the Harvard T.H. Chan School of Public Health (Department of Nutrition, Prof. Frank B. Hu, USA), and the Broad Institute (Metabolomic Platform, Prof. Clary Clish, United States). An annual International Symposium has been held for the last 10 years, each year on one side of the Atlantic, to discuss these findings. The 10th symposium took place at Tecnun (University of Navarra, San Sebastian, Spain) in June 2024.
Major findings on CVD risk in studies nested in PREDIMED (figure 1) include:
- –
2 branched-chain amino acids (leucine and isoleucine) were associated with higher CVD risk (stronger for stroke than for coronary heart disease) and this elevated risk was mitigated by the intervention with the MedDiet.39
- –
Short- and medium-chain acylcarnitines were associated with higher CVD risk, which was attenuated by the MedDiet interventions.40
- –
Plasma tryptophan levels were associated with lower CVD risk whereas kynurenic acid was linked to higher risk. The inverse association between the MedDiet intervention and CVD was attenuated by adjustments for changes in plasma tryptophan, suggesting a potential explanation for the protection provided by the MedDiet supplemented with extra-virgin olive oil.41,42
- –
Plasma glutamine levels were associated with lower CVD risk, whereas plasma glutamate predicted higher risk of both major CVD43 and HF, but not atrial fibrillation.44
- –
Reduced arginine availability, a precursor for nitric oxide, may limit its production. Higher global arginine availability, reflected by plasma levels of 3 urea cycle metabolites, such as [arginine/(ornithine+citrulline)], was associated with lower risk of CVD.45,46
- –
Several plasma ceramides were associated with higher CVD risk, which was attenuated by the MedDiet interventions.47 This discovery, which was subsequently replicated,48 can improve risk prediction.
- –
Plasma choline and the choline pathway (trimethylamine N-oxide, betaine, choline, phosphocholine, and α-glycerophosphocholine) were associated with a higher risk of major CVD events, HF, and atrial fibrillation.49,50
- –
Cholesterol esters were inversely associated with CVD risk. Shorter chain length and higher saturation of some lipids showed direct associations with a higher risk of CVD events. Polyunsaturated phosphatidylcholines (PC) and (PC)/lysoPC/PC-plasmalogens predicted lower CVD risk, whereas phosphatidylethanolamines, monoacylglycerols/diacylglycerols, and short-chain triacylglycerols showed a positive association with CVD.51–54
- –
Some metabolites of the citric acid cycle (Krebs cycle), specifically hydroxyglutarate, fumarate, and malate, were associated with higher CVD risk, improving risk prediction compared with the Framingham equation.55
Tartaric acid serves as an optimal biomarker for grapes and wine because it is found in equal quantities in both red and white wine, unlike polyphenols, which are up to 10 times less concentrated in white wine.36–38 In a substudy nested within PREDIMED, urinary tartaric acid has been used to assess the associations of moderate-to-light alcohol intake with CVD events. Additionally, tartaric acid inversely correlates with biomarkers of atherosclerosis and likely exerts anti-inflammatory properties.38,56–58(Poly)phenols may have bioactive effects depending on the individual's genetics and/or the ability of the microbiota to produce certain metabolites.59 The assessment of multiple urinary metabolites, using a novel method based on LC-MS, provided further evidence that urinary phenolic metabolites might confer strong protection against CVD.57,58 Further metabotyping approaches with dietary (poly)phenols may contribute to improving preventive cardiology from a precision nutrition perspective.59
The MedDiet and the gut microbiomeLong-term adherence to the MedDiet is associated with specific taxonomic and functional components of the gut microbiome.60 The protection conferred against CVD by the MedDiet is dependent on gut microbial composition, with a significantly stronger protective effect in individuals with a decreased abundance of Prevotella copri.61 However, understanding of the functional role of the gut microbiome in cardiometabolic disease remains limited. Inconsistent results are potentially related to small sample sizes, inadequate control of confounding factors, and variability in study design and analysis. Few studies have explored strain-level microbial features in relation to chronic systemic diseases, even though pathogenic mechanisms are potentially strain-specific rather than species-specific. MicroCardio was established in 2021 as an international microbiome consortium with standardized data processing and analysis examining the associations between metagenomes and T2D on a global scale and revealing robust cross-cohort microbial signatures in a strain-resolved manner.62 These advances provide reproducible analyses at both the community and strain levels, offering new mechanistic insights into the microbiome. Ongoing studies within the MicroCardio Consortium are currently investigating the relationships between healthy dietary patterns, the gut microbiome, microbial metabolites, CVD, and T2D.
Nonnutritive sweeteners and cardiometabolic riskDespite replacing sugar with nonnutritive sweeteners (NNS), the percentage of adults with obesity has steadily increased, potentially contributing to subsequent increases in CVD. The role of NNS requires re-evaluation. Initially, NNS were thought to be inert or even beneficial. However, they have been reported to induce microbiome-dependent glucose intolerance in mice.63 In particular, saccharin may potentiate glycan degradation pathways, a phenomenon also observed in the microbiomes of patients with obesity. In a randomized clinical trial, saccharin and sucralose induced harmful glycemic responses (restricted to the period of consumption).64 Longitudinal analyses using tensor factorization revealed significant temporal taxonomic and functional changes in microbiota associated with NNS intake.65 Germ-free mice conventionalized with microbiomes from participants with normal or elevated glycemic responses to NNS showed glycemic responses similar to those of their human donors. Variable effects of several NNS on glycemic responses have shown that some NNS might induce detrimental glycemic responses in a person-specific, microbiome-dependent manner. A key factor appears to be baseline microbiome composition. Further efforts in genetics and metabotyping are necessary to better understand mechanisms relating NNS intake to T2D and CVD risk.64,65
PREDIMED-Plus: energy-reduction and exercise added to the Mediterranean dietPREDIMED-Plus is a Spanish primary CVD prevention trial that randomized 6874 men and women with metabolic syndrome, aged 55-75 years, into 2 groups: one receiving a multidomain weight loss intervention, including an energy-reduced MedDiet, physical activity promotion, and behavioral support; and the other, a control group, receiving usual care and general recommendations on MedDiet without any caloric restriction. Recruitment started at the Navarre center in 2013. An additional 22 centers joined the trial during 2014-2015. All participants completed a 6-year intervention period in December 2022, and 2 additional years of observation were added. The trial ends in December 2024. The intervention group showed increased adherence to the MedDiet, lower body weight, and reduced CVD risk factors,66 as well as 3-year changes in total fat, lean mass and visceral fat in the expected direction.67 Several ongoing grants nested in PREDIMED-Plus can advance precision nutrition to better understand relationships between multi-omic signatures, the multidomain intervention, and disease.68
LIPIDS, METABOLITES AND CARDIOMETABOLIC RISKBlood lipids, lipid intake and cardiovascular disease: new avenues of researchCVD is the leading cause of mortality worldwide, while dementia ranks third in the US and Europe.69 Early-onset dementia can be detected through biomarkers linked to cardiovascular risk. Low-density lipoprotein cholesterol (LDLc) levels are associated with higher risks of dementia, whereas high-density lipoprotein cholesterol (HDLc) shows inverse relationships.70–72 Although low HDLc levels are risk factors for coronary heart disease, their causal relationship remains unclear, with inconsistent results in recent meta-analyses and Mendelian randomization studies.73–76 Improving HDL function may be more relevant for prevention than simply raising HDL concentrations. Dysfunctional HDL-c, such as decreased cholesterol efflux capacity, is associated with a higher CVD risk.71,77–80 In a matched case-control study nested within PREDIMED, low cholesterol efflux capacity, HDL particles with reduced antioxidant/anti-inflammatory capacity, and low levels of HDL-bound sphingosine-1-phosphate and ApoA-I were associated with a higher risk of coronary heart disease, regardless of HDL cholesterol levels and other risk factors.
Based on a systematic evaluation of the available evidence, the World Health Organization updated its recommendations on fat intake, advising reduced saturated fat intake, and encouraging its replacement with unsaturated fats, primarily to prevent CVD incidence.81 The certainty of these recommendations is considered low to moderate, due to limitations of the underlying studies. The specific effect of replacing saturated fat from dairy products, such as butter, with plant-based unsaturated fatty acids is subject to ongoing controversies.82–87 A recent project integrated data from different study types to generate more robust evidence on the quantitative effect of replacing saturated dairy fat with unsaturated plant fat.54 This work relied on lipidomics data from the participating studies. A randomized controlled trial that replaced saturated dairy fat with plant-based oils and spreads over several months was used to derive a multilipid score (MLS) reflecting diet-induced changes in 45 lipid metabolites. The application of the MLS to large population studies showed that these diet-related changes in the lipidome were associated with substantially lower risks of CVD and T2D. This study also used lipidomics data from PREDIMED to evaluate whether the benefits of the MedDiet intervention, which is high in plant-based unsaturated fat, depend on preintervention MLS levels. Notably, while no effect modification was detected on CVD risk, individuals with initially adverse MLS showed the greatest reduction in T2D risk in response to the MedDiet intervention,54 strengthening the evidence for a causal effect of replacing saturated dairy fat with unsaturated plant fats on reduced cardiometabolic risk. Additionally, specific results from PREDIMED suggest that lipidomics profiling could be valuable in tailoring dietary fat interventions to the most at-risk groups.
HDL, microRNAs and nano-discs in cardiovascular preventionMicroRNAs (miRNAs) are small noncoding molecules that regulate messenger RNA function by hybridization.88 They are emerging as possible biomarkers and pharmacological targets. In fact, circulating miRNAs have been described as associated with CVD incidence in prospective human studies.89,90 Interestingly, the miRNA-HDL profile of patients with familial hypercholesterolemia differed from that of a control group.91 Furthermore, infusions of certain miRNAs can prevent plaque formation, and silencing miRNA-33 is a promising strategy for tackling atherosclerotic CVD.92
As a proof of concept, the design and development of a synthetic high-density lipoprotein (sHDL) nanoparticle, incorporating a locked nucleic acid antagonist targeting a candidate miRNA have been successfully completed at Hospital del Mar Research Institute, Spain, under the leadership of Dr M. Fitó. Tailored effects of these nano-discs were observed in in vitro models regarding cholesterol efflux capacity in macrophages and the excretion of pro-inflammatory molecules in endothelial cell lines, by silencing a candidate miRNA using an antagonist in agreement with previous suggestive findings.93,94
Dairy products, metabolomics and cardiometabolic diseaseDifferent food groups are currently being studied to identify metabolomic signatures and their associations with CVD or T2D. For example, the evidence from subjective dietary assessments of dairy consumption is mixed and inconsistent. Fermented dairy appears to have an inverse relationship with T2D, while total dairy shows no association.95 Some studies reported a positive or no association between butter and CVD, but an inverse relationship with T2D. Objectively measured fatty acids of dairy fat showed inverse associations with T2D, which were also confirmed in metabolomics analyses using 82 metabolites identified in the UK-based Fenland and EPIC-Norfolk cohorts.96,97
Red meat, metabolomics and cardiometabolic diseaseRed meat intake has been consistently associated with a higher risk of noncommunicable diseases, including T2D98 and CVD.99 A combination of metabolites is more effective in categorizing dietary habits than individual metabolites.100 Plasma metabolomic biomarkers underlying the association between heme iron intake and T2D are likely to provide strong pathophysiological support for the harmful association between red meat intake and cardiometabolic disease.100 Heme iron intake was recently found to account for >50% of the association between unprocessed red meat intake and the risk of T2D, and some plasma metabolites, including L-valine and uric acid, were identified as potentially mediating the heme iron-T2D relationship.101
Metabolites, mortality, and longevityMultimetabolite predictors of mortality risk and longevity can provide further insights into the mechanisms behind these factors.102,103 In 4 prospective cohorts, a healthy lifestyle (not smoking, a healthy weight, regular exercise, a high-quality diet, and moderate alcohol consumption)104 was reflected in a metabolomic signature showing that these healthy habits can increase life expectancy by up to 14 years after the age of 50 years.105 In addition, in 11,634 US participants from the NHS/NHS2/HPFS and 1878 participants in PREDIMED, 75 metabolites were positively associated with all-cause mortality while 32 were negatively associated with all-cause mortality.103 Specifically, triacylglycerols with longer and less saturated chains were linked to increased longevity. Differences in levels of nucleosides, amino acids, and several lipid subclasses also predicted long-term mortality.
SUMMARY AND FUTURE RESEARCH DIRECTIONSRecent advances in omics technologies and machine learning methods have accelerated precision nutrition and cardiometabolic disease research. Metabolomics analyses have greatly contributed to the prevention of cardiometabolic diseases by enhancing our understanding of biochemical pathways and mechanisms. The extended follow-up of PREDIMED for mortality (up to 2019) will be instrumental in identifying multimetabolite signatures associated with long-term total and cardiovascular mortality in high-risk patients. The PREDIMED-Plus study will examine the effects of the multidomain intervention on T2D after 7 years of follow-up, as well as chronic kidney disease.106 CVD (the primary endpoint) will be assessed after 8 years. The rich collection of dietary and lifestyle data, biological samples,107 and metabolomics data will enable further insights into the metabolic pathways underlying diet and cardiometabolic diseases.68
Integrating metabolomics in multiple body fluids in large trials and cohort studies using hard clinical events will deepen our understandings of mechanisms at the systems level.1 This approach will help to design more effective Mediterranean-style dietary interventions to improve cardiometabolic health. The symposium highlighted future directions in precision nutrition research, including: a) the development of more robust metabolite biomarkers for predicting long-term cardiometabolic disease risk and mortality; b) the inclusion of more diverse populations and dietary patterns from different cultures; and c) the need for enhanced translational research to bridge the gap between nutrition science and clinical applications.
FUNDINGThe PREDIMED trial was funded by the Spanish Ministry of Health (Instituto de Salud Carlos III [ISCIII], through the PREDIMED Network grant RD 06/0045, 2006–2013, coordinated by M.A. Martínez-González; and a previous network grant RTIC-G03/140, 2003–2005, coordinated by R. Estruch). Additional grants were received from the Ministerio de Economía y Competitividad-Fondo Europeo de Desarrollo Regional (Projects CNIC-06/2007, CIBER 06/03, PI06-1326, PI07-0954, PI11/02505, SAF2009-12304) and by CIBER Fisiopatología de la Obesidad y Nutrición (CIBERobn). In addition, metabolomic profiling studies nested in the PREDIMED trial were funded by NIDDK and NHBLI grants (R01HL118264, R01DK127601, R01DK102896, and U2CDK129670).
The PREDIMED- plus trial (2014-2019) was initially funded by the European Research Council to M.A. Martínez-González (https://cordis.europa.eu/project/id/340918/es, accessed on September 6, 2024). Metabolomic profiling studies nested in the PREDIMED-Plus trial were funded by a NIDDK grant (1R01DK127601), and the official Spanish Institutions for funding scientific biomedical research, CIBER Fisiopatología de la Obesidad y Nutrición (CIBEROBN) and Instituto de Salud Carlos III (ISCIII), through the Fondo de Investigación para la Salud (FIS), with 6 coordinated FIS projects led by J. Salas-Salvadó and J. Vidal, including the following projects: PI13/00673, PI13/00492, PI13/00272, PI13/01123, PI13/00462, PI13/00233, PI13/02184, PI13/00728, PI13/01090, PI13/01056, PI14/01722, PI14/00636, PI14/00618, PI14/00696, PI14/01206, PI14/01919, PI14/00853, PI14/01374, PI14/00972, PI14/00728, PI14/01471, PI16/00473, PI16/00662, PI16/01873, PI16/01094, PI16/00501, PI16/00533, PI16/00381, PI16/00366, PI16/01522, PI16/01120, PI17/00764, PI17/ 01183, PI17/00855, PI17/01347, PI17/00525, PI17/01827, PI17/00532, PI17/00215, PI17/01441, PI17/00508, PI17/ 01732, PI17/00926, PI19/00957, PI19/00386, PI19/00309, PI19/01032, PI19/00576, PI19/00017, PI19/01226, PI19/00781, PI19/01560, PI19/01332, PI20/01802, PI20/00138, PI20/01532, PI20/00456, PI20/00339, PI20/ 00557, PI20/00886, and PI20/01158. Other funding for PREDIMED-Plus included the Especial Action Project entitled: Implementación y evaluación de una intervención intensiva sobre la actividad física Cohorte PREDIMED-Plus grant (J. Salas-Salvadó); the Recercaixa grant (2013ACUP00194) (J. Salas-Salvadó); and grants from INSA-María de Maeztu Unit of Excellence (grant CEX2021-001234-M funded by MICIN/AEI/FEDER, UE).
STATEMENT ON THE USE OF ARTIFICIAL INTELLIGENCENo artificial intelligence applications were used to write this manuscript.
AUTHORS’ CONTRIBUTIONSM.A. Martínez-González wrote the first draft. All authors provided substantial contributions to the conception and design of the work, the acquisition, analysis, and interpretation of data for the work, revised the manuscript critically for important intellectual content and gave their final approval of the version to be published. All coauthors agreed to be accountable for all aspects of the work in ensuring that questions related to the accuracy or integrity of any part of the work are appropriately investigated and resolved.
CONFLICTS OF INTERESTM. Guasch-Ferré acknowledges that The Novo Nordisk Foundation Center for Basic Metabolic Research is supported by an unrestricted grant from the Novo Nordisk Foundation (grant no. NNF18CC0034900). J. Salas-Salvadó expresses appreciation for financial backing from ICREA through the ICREA Academia program. O. Castañer acknowledges ISCIII contracts CP21/00097, PI20/00012, and the CIBER Network of Epidemiology and Public Health (CIBERESP). P. Mena received funding from the European Research Council under the European Union's Horizon 2020 research and innovation programme (PREDICT-CARE project, grant agreement No 950050). F.J. Planes was supported by the European Union's Horizon 2020 research and innovation program (STANCE4HEALTH, Grant No. 816303). All other authors declare no conflict of interest.
We thank Andrés Moncada-Parra for his collaboration in the preparation of the graphical abstract and other material tasks for the collection of information used to prepare the first draft of the manuscript.